Title:
PINN modeling for GaN hotspot prediction
Students:
Description:
The application of ML to RF device modeling and failure prediction has been limited in literature, in part, because of the limited amount of available data. Common ML efforts use large experimental data sets to train ML algorithms such as neural networks. In academic research initiatives, studies often report data on less than ten power or RF devices, which is an insufficient amount of data to train purely data-informed ML algorithms. However, multi-physics modeling via FEA is commonly applied when analyzing experimental data and making device design choices. In the zero-data limit, ML algorithms can be trained exclusively on these physical models resulting in PINNs. After training, these PINNs can provide a virtually instantaneous solution to the partial differential equations (PDEs) governing electrothermal transport over vast parametric spaces. Moving away from the zero-data limit, hybrid ML algorithms can be constructed which are trained on both multi-physics models and experimental data. In the hybrid approach the algorithm is trained initially on known physics as a baseline. Therefore, the amount of experimental data required for training is expected to be dramatically reduced. Most importantly, the ML algorithm isn’t bound by the rigid mathematical framework behind FEA models. Therefore, phenomena not explicitly contained in the governing equations, such as failure mechanisms, can be learned.
Pictures:
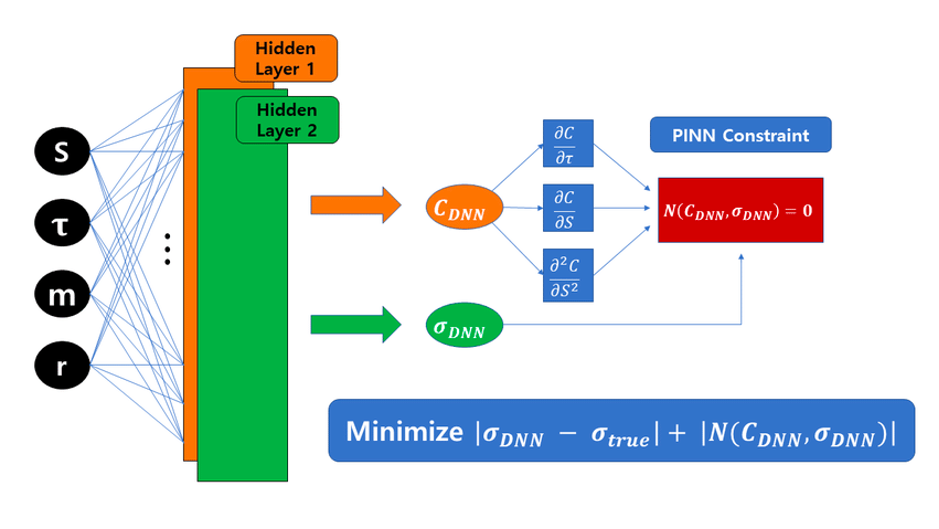